Professor

William Jefferys
Office: RLM 16.236
Hours: TBA
Phone: (512) 471-1455
bill@clyde.as.utexas.edu
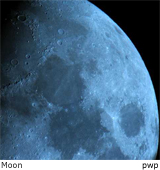
|
 |
This is a course in Bayesian statistics. The instructor is an
astronomer by profession, so the course will emphasize applications
to the physical sciences; however, the material of the course will be
useful for applying Bayesian inference in a wide variety of contexts.
Bayesian inference is a powerful and increasingly popular statistical
approach, which allows one to deal with complex problems in a
conceptually simple and unified way. The recent introduction of
Markov Chain Monte Carlo (MCMC) simulation methods has made possible
the solution of large problems in Bayesian inference that were
formerly intractable. This course will introduce the student to the
basic methods and techniques of modern Bayesian inference, including
parameter estimation, MCMC simulation, hypothesis testing, and model
selection/model averaging in the context of practical problems.
Books
Data Analysis: A Bayesian Tutorial (D. S. Sivia, Oxford University Press),
Measuring Uncertainty: An Introduction to Bayesian Inference (Samuel
Schmitt, Addison-Wesley, to be reprinted as a course packet, obtain
at Texas Union).
Optional: Bayesian Statistics (Gelman et. al.)
Topics
Not necessarily in this order; subtopics will be presented as
appropriate
Review of probability calculus. Interpretations of probability (e.g.,
frequency, degree-of-belief). Coherence. Bayes's Theorem. Joint,
conditional, and marginal distribution. Independence. Prior
distribution, likelihood, and posterior distribution. Bayesian
estimation and inference on discrete state spaces. Likelihoods, odds
and Bayes factors. Simple and composite alternatives.
Prior selection. Subjective and objective priors. Priors as a way to
encode actual prior knowledge. Sensitivity of the posterior
distribution to the prior. Hyperpriors (priors on priors) and
hierarchical Bayes models.
|